Too many questions remained unanswered
I was very excited to upload my data and get new insights from my datasets. But the results I got disappoint me very much.
The first data set contained summarized data, mean values of the items in my survey, differentiated by occupational group. I knew this was not the ideal basis for an analysis by Chartpixel, but the key-insight got it completely wrong. High values in my data represented a risk, low value a secure environment. But the “key-insights” by the AI interpreted high values as positive, low values as negative. There is no input option to tell Chartpixel how to interpret the values. This is why the automatic analysis, immediately after uploading the data, came to the wrong conclusions.
Furthermore, Chartpixel seems to read causal relationships from my data, which it does not contain at all. I only have one measurement point in my data and yet the AI formulates that one variable predicts the values of the other, i.e., that it is a predictor. Unfortunately, I cannot find any information in the evaluation or in the charts as to which statistical analyses lead to this statement. There is therefore no way of understanding the results even from a math point of view.
I have uploaded a second data set that contains raw data from 90 people. Even though the data set is rather small, it should now be data that Chartpixel can process reliably. This is what the support team said in response to my feedback after the first attempt.
Although I had uploaded a German-language data set and the output language is set to German, I received all item descriptions in the charts in English. The meaning of the item names had been changed by the translation.
One statement in the “key insights” of this evaluation was: “Time Management leads the self-assessment of work quality.” Apart from the fact that the term time management does not even appear in my data set, the content of this statement is incomprehensible to me.
Again, there is no explanation as to how the AI arrived at this result and which items from my questionnaire were included in the variable “time management”, which was presumably constructed by the AI.
Apart from the fact that the content of the statement is incomprehensible to me, I would have no way of explaining to my listeners, to whom I present the results, how these conclusions were drawn from the data. In my view, there is a lack of transparency in the calculations and this leads to a lack of my trust in the calculations and interpretations.
I could mention other points that seem strange to me in Chartpixel's evaluation of this data set, but I don't want to make the text too long.
My conclusion is that the high expectations I had after reading the very positive reviews were not fulfilled. For the kind of data, I want to analyse, e.g., from employee surveys, Chartpixel seems unsuitable. I will ask for a refund.
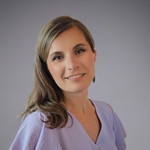
Andrea_ChartPixel
May 6, 2025Thank you so much for your honest and detailed feedback. I’m truly sorry ChartPixel didn’t meet your expectations, and I appreciate the time you took to share your experience with us.
What data can ChartPixel handle?
ChartPixel is designed to provide automatic insights and visualizations from raw tabular data. It’s powered by machine learning models, statistical tests, and data aggregations to help with the chart creation process. The more detailed and granular your data, the better ChartPixel can deliver useful results. To help users to get started, we provide an upload guide in your workspace, along with blog posts and tutorials, and we emphasize in our welcome email that uploading raw tabular data is the best way to unlock ChartPixel’s full potential.
On the other hand, summary tables or “pivoted” data (where values are already aggregated, such as averages by group with an index across columns) often don’t provide enough detail for meaningful statistical inference or AI-driven insights. ChartPixel is positioned in the similar space as traditional statistical tools like SPSS, R, and Stata, but it is made to be more approachable and beginner-friendly, focusing on visualization and ease-of-use. Like those tools, uploading summary tables or using them for predictive modeling will not yield reliable inferences. The most insightful analysis always comes from working with raw, individual data points.
Can I still use ChartPixel with summarized data?
While ChartPixel is optimized for raw, row-level data, you can still use it for quick visualizations with summarized tables or pivot data, especially if you’re not looking for deep analysis or automated insights. Many users like the ability to quickly turn their summarized data into clear, charts - saving time and simplifying the process, rather than building charts manually in Excel or other programs.
On transparency and explanations
We fully understand how important it is to know how ChartPixel generates its analyses. For summarized or small datasets, ChartPixel intentionally limits the depth of analysis and disables certain analysis tools (by greying the buttons out). Each chart provides an “insight quality” metric from 0 to 5 (the higher, the better).
Charts created from summary tables usually receive a zero score with a warning, making it easier for you to judge when results may need extra caution. When you upload raw, granular data, you have access to our full suite of analysis tools—just click the analysis icon at the bottom of your chart to run further statistical tests (such as trends or significance tests) and view detailed results, complete with clear interpretations.
About translations
We’re genuinely sorry for any confusion the translation or automatic insights may have caused. The language feature only went live a couple of days ago during the campaign, and we are still refining it. ChartPixel can automatically rename long column names to keep charts readable. For example, a long survey question like “Can you please name the single most important reason for purchasing a second mobile phone?” might be changed to “Top Reason for Second Phone Purchase.”
When processing long column names in languages other than English, the algorithm attempted a translation with the renaming, which we actively working to improve. If you would like, you can easily disable this in your project settings by toggling off “Rename long column names.”
Choosing the right tool for your job
While ChartPixel is designed to make exploring and visualizing large, raw datasets easier and faster, it is not always the right fit for every scenario, such as already summarized or pivoted tables that require detailed inference. For summarized tables, there are excellent alternatives, like Flourish for creating stunning charts from summary data, or Datawrapper for easily sharing charts online.
If you need infer insights from summarized tables, you might also try asking a large language model like ChatGPT or Claude. However, these options aren’t ideal for handling very large datasets either and require more prompts and carefully crafted context.
Although we could go down that path and extend functionality for summary data, it’s not part of our current roadmap to process your raw data through third-party tools (which is also known as wrapping). We strive to offer a productive, reliable platform for visualizations grounded in statistical analysis, allowing you to explore your data quickly and glean actionable insights and especially from more complex datasets. Next on our roadmap is adding more interesting statistical test, new chart types and the possibility to add more context to iron out unexpected results. Ultimately, we want ChartPixel to be as helpful as possible for the right use case.
Thank you again for your thoughtful feedback. It truly helps us make ChartPixel better for everyone and clarify the use cases for our customers.